A to C
A
Absolute benefit
Difference between the untreated/control risk or rate (respectively individual/group-average prediction, or event on an observed group) and the corresponding treated risk or rate in the same individual(s) or groups, the former being referred to as Rc and the latter as Rt.
For patient i, the Absolute Benefit ABi is expressed as follows:
ABi=Rc,i−Rt,i
See also Number of Prevented Events, Effect Model law
Activity (drug)
Physiological response that a drug produces.
Acute disease
Disease for which the time span between its beginning and the unwanted clinical outcome can be quantified within an order of magnitude of hours to days.
Adverse drug reaction (ADR)
Noxious and unintended response to a drug, that may result from multiple sources (e.g. from the authorized use of a medicinal product at normal doses, from medication errors, from drug-drug interaction, and others).
See also ADR classification by World Health Organisation (p4)
Affinity
Ability of one biological compound to combine with another compound and/or their strength of binding (i.e. a ligand that combines with its receptor).
The affinity corresponds to the association constant KaKa of the reaction
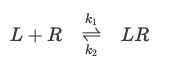
calculated by the formula according to the mass action law.
Agonist
Ligand that upon binding to a receptors triggers a physiological response. Agonist binding to a receptor can be characterized both in terms of efficacy and affinity. Agonists can be full, partial or inverse.
See also agonist (wikipedia).
Allometry
Study of the relationship between morphological, physiological and ecological characteristics (e.g. heartbeat frequency) of different individuals or species scale with their size (often characterized with weight).
See also: jinko allometry module
Allosteric modulators
Biological compound that binds to a receptor at a site distinct from the active site and which indirectly influences the effect of an endogenous ligand at the receptor. It commonly induces a conformational change in the receptor, which alters the affinity of the ligand for the receptor. Positive allosteric modulators increase the affinity, whilst negative allosteric modulators decrease it.
Annotation
Any data that characterizes a piece of knowledge for the sake of using it in modeling (e.g. parameter average value, strength of evidence, variability, connecting entities).
Antagonist
A (receptor) antagonist is a ligand that binds to a receptor but does not activate a physiological response as an agonist does. Because of this lack of activation, antagonists have a measurable affinity for their cognate receptors but no efficacy. As antagonists compete with another ligands for receptor occupancy they are able to achieve receptor blockade, either by inhibiting the binding of agonists or by inhibiting the function of agonists at the receptor. Antagonists can be competitive or non-competitive, each of which can be reversible or irreversible: + A competitive antagonist binds to the same site as the agonist but does not activate it, thus it blocks the agonist's action by competing with the agonist for the receptor. The action of a competitive antagonist can be overcome by increasing the dose of the agonist. + A non-competitive antagonist binds to an allosteric site on the receptor (i.e. a different site than the agonist binding site) to prevent activation of the receptor. The action of a non-competitive antagonist cannot be overcome by increasing the dose of agonist. + A reversible antagonist binds non-covalently to the receptor, therefore can be replaced by competing ligands in a concentration dependent manner or washing. + An irreversible antagonist binds covalently to the receptor and cannot be replaced by either competing ligands or washing. The effect of irreversible antagonisms can only be reversed by synthesis of new receptors.
See inhibitors.
Applicability Domain
The Applicability Domain (AD) is and defines the specific role and scope of standalone model. This definition mirrors the concept for CoU for submodels but also apply to combined model. It briefly describes the main specs of a model allowing to instantly grasp its composition, breadth, width and limitations. The AD is typically a single sentence based on a fill-in-the-gaps template.
Assertion
The linguistic definition of an assertion is rooted in the philosophy of language. Generally it is an act of speech in which something is claimed to hold, for instance that "there are infinitely many prime numbers". As knowledge is often organized around claims with direct and indirect evidence supporting them, assertions can be regarded as the units of knowledge communicated between humans.
Nova specifies its definition in Knowledge Modeling where assertions are the principal pieces of knowledge carrying scientific information in the form of plain language that will be assembled to a Knowledge Model
Assumption
An assumption is generally a thing that is accepted as true or as certain, without proof.
More precisely in context of knowledge-based modeling, an assumption usually circumvents unknown or uncertain biological functioning (knowledge gaps) though e.g. analogy, extrapolation or the use of a less granular description (simplification).
B
Beta-(sub)model
First version of an integrated Computational (Sub)Model which connect all inputs and outputs that are expected in the final version. The included bioreactions are phenomenological and do not need to be exhaustive as they will eventually be reworked. They should be as simple and minimal as possible, even if this means directly connecting variables or hard-coding mechanisms.
Bias
In a statistical sense, bias is a systematic distortion of a statistical result due to a factor not allowed for in its derivation. Bias can result from several sources: one-sided or systematic variations in measurement from the true value (systematic error); flaws in study design; deviation of inferences, interpretations, or analyses based on flawed data or data collection; etc.
Of importance are: +bias in clinical trials, which are systematic errors that encourage one outcome over others. The potential effect of bias is that investigators will come to the wrong conclusions about the beneficial and harmful effects of interventions. + publication bias is a type of bias that occurs in published academic research. It occurs when the outcome of an experiment or research study influences the decision whether to publish or otherwise distribute it. Publishing only results that show a significant finding disturbs the balance of findings, and inserts bias in favor of positive results.
For more detailed information about bias in clinical research see (this paper)
Bioavailability
In pharmacology, bioavailability is the fraction of an administered dose of unchanged drug that reaches the systemic circulation. By definition, when a medication is administered intravenously, its bioavailability is 100%. Bioavailability is also defined as the extent and rate at which a drug is absorbed into a living system and is made available at the site of physiological activity. It is one of the principal pharmacokinetic properties of drugs, a subcategory of absorption that is measured in Phase 1 and Phase 2 clinical trials and must be considered when calculating dosages for non-intravenous routes of administration.
Biological entity
A biological entity refers to any biologically active unit (such as a specific kind of molecule - e.g. ion, protein - aggregate of molecules or cell) represented in a Knowledge Model of Computational Model. Usually only those entities (other than in e.g. genome-wide models) mainly those entities known for playing a role in the pathophysiology of a disease of interest are susceptible of being represented in one of Nova's models.
Biomarker
In a medical sense a “biomarker” (“biological marker”), refers to a broad subcategory of medical signs – that is, objective indications of medical state observed from outside the patient – which can be measured accurately and reproducibly. For a more complete definiton see this paper.
Biomarkers (in Computational Models of disease and treatment may be classified as follows:
- A prognostic biomarker is a biological entity or signal which measurement is correlated with the disease outcome
- A diagnostic biomarker is a biological entity or signal which measurement is correlated with the disease occurrence
- A theranostic biomarker is a biological entity or signal which measurement is correlated with a treatment's resulting absolute benefit
Biomarkers predictive/diagnostic values should be proven through appropriate clinical studies/trials. One should make the difference between correlation and predictive values. The latter measures the potential utility in medical practice of the biomarker.
Biomarkers, especially theranostic biomarkers, are often mixed up with surrogate markers (used as primary measures of the effectiveness of investigational drugs in definitive drug trials). In a regulatory context, surrogate marker, need to be validated before they can get used. Validated surrogate markers are those for which evidence has established that a drug-induced effect on the surrogate predicts (results in) the desired effect on the clinical outcome of interest (for example survival). There are also "unvalidated surrogates". These “reasonably likely” predict the clinical benefit of interest, but for which there is insufficient evidence to establish that such an effect, does, in fact, result in the desired clinical outcome (for more info see this FDA perspective).
C
Calibration
Calibration is a process in which a priori unknown and estimated parameters are refined through a numerical procedure, more specifically optimization routines in order to achieve a desired behavior of the model.
While the term "parametrization" may be used to mostly human curation of sources for parameter values, "calibration" denotes an automated numerical procedure where a number of parameters is usually calibrated in parallel. As opposed to single criterion optimization (where some error metrics may simply be used), complex calibration problems often need to establish compliance with a heterogenous dataset representing the bounds and targets of desired model. The inclusion of such dataset requires a somewhat uniform representation with "scorings" Scoring. In practice, these scorings are used in linear combinations to construct the objective function used to minimize or to maximizing parameter likelihood, depending on the algorithm used for the calibration.
Chronic disease
A disease that persists for a long time. A chronic disease is one lasting 3 months or more, by the definition of the U.S. National Center for Health Statistics and for which the time span between its beginning and the unwanted clinical outcome can be quantified within an order of magnitude of years to decades. Chronic diseases generally cannot be prevented by vaccines or cured by medication, nor do they just disappear.
See also Acute Disease
Clinical
Refers to anything done by a physician when interacting with a patient. It used to refer to medical work that relates to the examination and treatment of ill people.
Clinical outcome
Clinical outcomes are measurable changes in health, function or quality of life that can be perceived by a patient. Constant review of clinical outcomes establishes standards against which to continuously improve all aspects of medical practice.
Clinical score
A standardized quantitative indicator of the seriousness of a disease, for a particular patient, at a particular instant in time. The clinical score is obtained on the basis of a series of clinical and/or biological and/or imaging and/or pathological factors assessed by a physician at the bedside, or with lab results. For example a clinical score can be used to predicts mortality of a given patient.
Clinical submodel
Refers to the submodel which binds the disease process (derived from the disease model) with a clinical outcome.
Combined targets
A single drug with several targets.
Compartment
A compartment is a word used to represent a specific subsection or structure of the body in a computational model. The level of granularity really depends on the Context of Use and of the submodels making up the whole model. Examples can be : Mitochondria, Epithelial Cell, Lungs, Liver
Computational model
Mathematical model to study the behavior of a complex system by computer simulation. The system under study is often a complex nonlinear system for which simple, intuitive analytical solutions are not readily available.
Confounding factors
A confounding factor in a study is a variable which is related to one or more of the variables under investigation in this study. A confounding factor may mask an actual association or falsely demonstrate an apparent association between the study variables where no real association between them exists. If confounding factors are not measured and considered, bias may result in the conclusion of the study.
Constitutive activity
Molecule activity which exists in the absence of a bound ligand. It may be blocked by an inverse agonist.
Context of use
A statement that defines the specific role and scope of the computational model used to address the question of interest.
Control arm
In a clinical trial, a group of patients can be part of the control arm meaning that they are not given the actual drug/intervention under study. They can take the standard, a placebo or nothing. The interest of this control arm is to compare the outcomes with the experimental arm in term of safety and effectiveness.
Control (base) rate
Cumulative hazard rate of an individual without treatment. It appears in the Effect Model law under its instantaneous index called Rc.
Control (base) risk
Risk of event (outcome) in untreated individual(s) in the context of a prediction. It is cumulative over time. The corresponding instantaneous index is the treated hazard Rc(t)Rc(t).
CRO
CRO refers to Contract Research Organization which is a company contracted by a pharmaceutical and biotech industry to manage and lead the company's trials, duties and functions. Contract research organizations manage almost all aspects of a clinical trial, from site sand patient selection through regulatory aspects and particularly the approval from the FDA.